Columbia University “Machine Learning in Science & Engineering” Conference
08 December 2020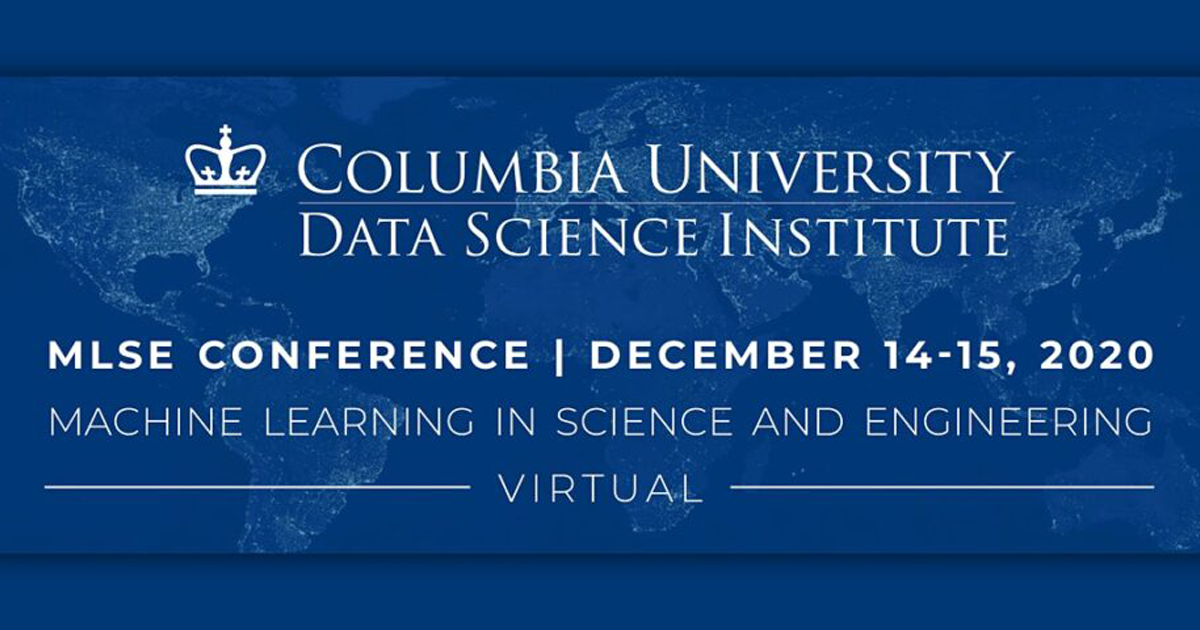
Eleven dedicated tracks will highlight innovations from a diverse range of disciplines and practices. MLSE 2020 will bring together over a thousand attendees from academia, government, and industry, as well as over a hundred speakers and dozens of research teams from national and international universities and organizations.
Florence d’Alché-Buc, Professor at Télécom Paris, Institut Polytechnique de Paris, will give a keynote address at MLSE 2020 in the Biology Track, as part of the cooperation between Columbia University and IP Paris within the Alliance Program. This cooperation will now have new horizons following the creation of IP Paris’ new interdisciplinary center on artificial intelligence, “Hi! Paris”.
Florence d’Alché-Buc’s current research interests are on machine learning, network inference, structured prediction, and dynamical system modeling. She was previously a researcher at Genopole and still retains a great interest for bioinformatics and medical applications. In her talk, she will present ongoing work [1] on structured prediction with operator-valued kernels for solving a problem linked to metabolite identification.
Metabolite identification from tandem mass spectrometry data has recently attracted interest in the machine learning community. This particular problem can be stated as a supervised learning task where the input variable is a mass spectrum and the output variable is a molecule, e.g. a labeled graph. A family of kernel-based approaches, called Input Output Kernel Regression (IOKR), developed at Télécom Paris in collaboration with Aalto University and INRAE, has shown excellent performance in solving this problem as a structured prediction.
Follow Florence d’Alché-Buc in the Biology Track Session 3 on Monday, December 14 at 2:30 pm (GMT -5, French time: 20h30).
*Registration mandatory – free registration for students and post-docs; paid registration for others
[1] Kernel-based Structured Prediction for Metabolite Identification, Florence d’Alché-Buc, Télécom Paris, Institut Polytechnique de Paris; Céline Brouard, INRAE; Luc Brogat-Motte, Télécom Paris, Institut Polytechnique de Paris; Alessandro Rudi, INRIA Paris; Juho Rousu, Aalto, Helsinki.