Machine learning to predict French residential buildings' energy performance
17 April 2023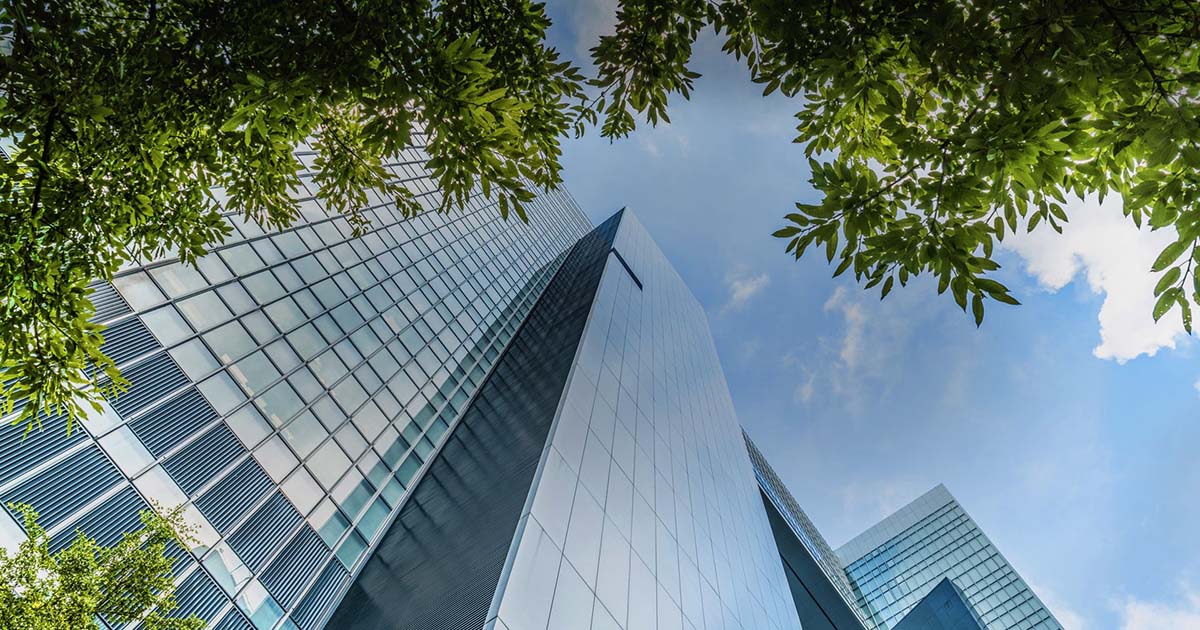
The goal of the model is to improve the measurement of the climate performance of financial institutions’ mortgages portfolios. The major change from most existing approaches is the prediction of both labels (Energy Consumption and GHG Emissions) parallelly, and without recourse to the Energy Performance Certificates (EPCs) intrinsic variables (variables needed for the EPCs labels closed-form formula). To date, very few papers describe such a data-driven approach to predict French EPCs. We tested our models on a sample dataset of physical features of the buildings to evaluate the potential of such an approach. The results were positive and will be the core of our future work.
Header image source Smart Impulse