AI Explainability at the IHM Conference 2022 at UNamur: Misdirection of XAI from technical solutions to user adaptation
30 April 2022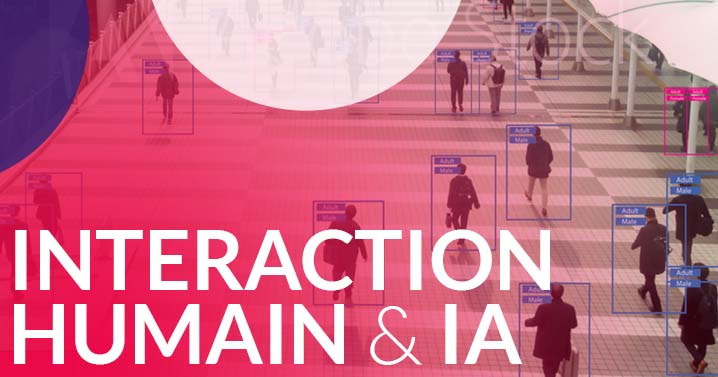
On the first day, I attended the workshop on AI Explainability that brought together researchers from both the HCI and Computer Science communities.
The workshop was opened by UNamur professors Bruno Dumas, specializing in HCI, and Benoît Frénay who works on Machine Learning. Dr Frénay presented the XAI research field and the interdisciplinary research being conducted at UNamur on this topic. He pointed out the lack of a user-centered approach in the XAI machine learning community where less than 1% of accepted papers in major conferences, such as NeurIPS, test their XAI methods with user studies.
The rest of the morning was devoted to the presentation of eight abstracts, including mine, related to XAI research with either a computer science or HCI angle. My presentation focused on a user study in the insurance domain to test an explainability interface. In this study, conducted with the French regulator of financial services, the ACPR, we designed an interactive XAI system with the aim to trigger curiosity within non-expert users to incite them to pay more attention to explanations of the algorithm. Other presentations ranged from a technical point of view to XAI – such as Julien Delaunay’s APE: Adapted Post-Hoc Explanations that switch from local feature-based explanations to rule-based ones when appropriate – to more user-oriented approaches, namely Rebecca Marion’s approach. Her work proposed a visualization method to improve user’s understanding of a dimensionality reduction algorithm, Multidimensional Scaling (MDS), that is widely used in the social sciences.
To summarize this morning in one point, there is a significant lack of HCI approaches in XAI. There are few user studies in technical works, and the few that do exist lack rigor and consideration of user needs. We need more user-centered work and we need to make sure that technical approaches start from genuine user needs, such as ontology-based approaches (presentations by Sarah Pinon and Grégoire Bougoin) that mimic human reasoning processes.
In the second part of the XAI workshop, we had rich discussions on four themes: “actionability” (or controllability), “user profiles”, “from modelisation to representation”, and “evaluation of XAI”. The organizers are currently preparing a summary of these brainstorming sessions.
Just as this conference opened with explainability, it also closed with this theme with Prof. Katrien Verbert’s keynote. Prof. Verbert gave an overview of the wide range of projects she leads in the Augment HCI team of KU Leuven. Her team explores various domains of applications of XAI including recommendations for music, educational exercises, agriculture with a Grape Quality Predictor, human resources, and nutrition… She also published numerous papers in top conferences on the design and visualization of explainability systems that usually involve user studies. Through her work, she demonstrated the need to adapt explanations to user types including their domain expertise and need for cognition, to involve end-users in the design of the XAI systems to better meet their needs, and to carefully balance insight, controllability and information overload.
Perhaps one of the most striking moments of the conference for me was Wendy McKay‘s comments after Prof. Verbert’s presentation, who couldn’t help but notice how the field of XAI starts from solutions to user needs when it should be the other way around. The field of XAI started with computer scientists who were trying to develop computational methods to bring transparency to their AI tools. The user-centric culture was absent from the beginning. It is only now starting to emerge, but since technical solutions already exist, it has been difficult for XAI researchers to ignore them completely and start from a blank page as is required for a user-centric designed approach. Yet, this will nevertheless be necessary as we crucially need to find explanations that are appropriate for each context and user.
All the presentations from the workshop on explainability are available here.