Interpretability, Accountability and Robustness in Machine Learning
16 February 2021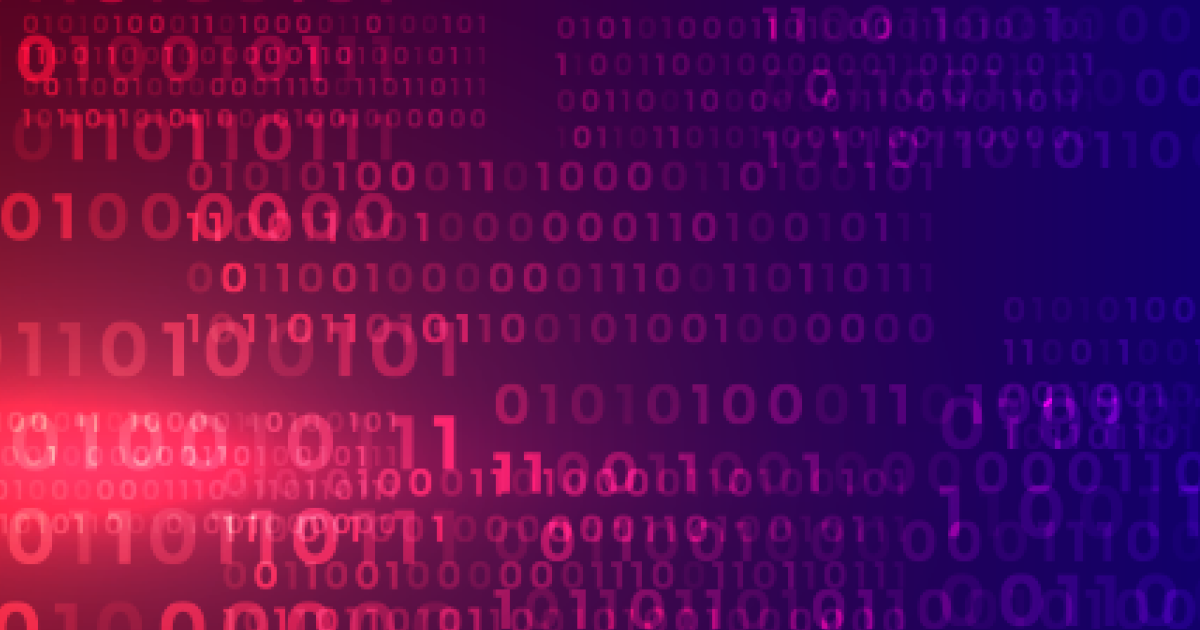
There are two main types of approaches to achieve explainability of the algorithms:
- Approaches that explain decisions for the existing models.
- Approaches which modify the model and/or its training process by incorporating the ability to explain.
Methods from the first group are in place when the AI algorithm is fixed and they give insights in why certain output has been produced for the given input.
Approaches of the second group change the design of the algorithm to produce explanations together with the predictions or force the algorithm to produce explainable solutions.
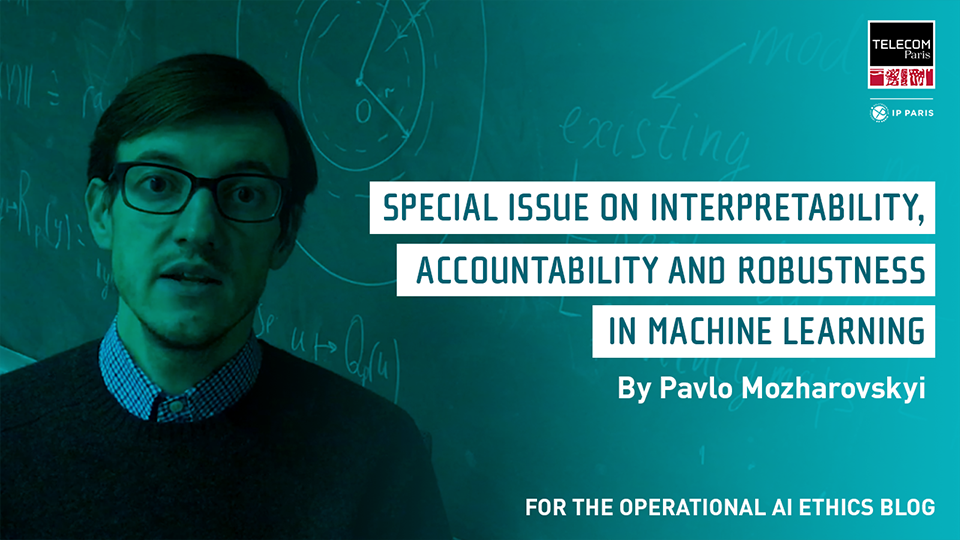
_____________________________________________________________________
By Pavlo Mozharovskyi, Télécom Paris – Institut Polytechnique de Paris
_____________________________________________________________________
Illustration: Designed by starline / Freepik