Human–where–in the loop?
24 March 2023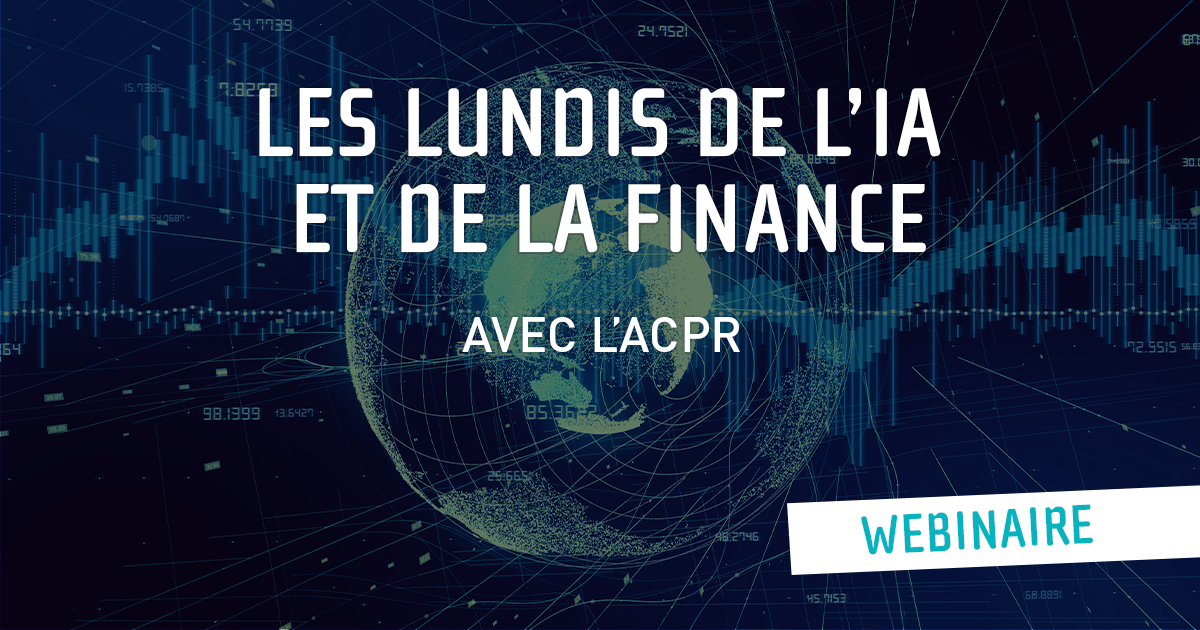
David Bounie, professor of economics at Télécom Paris, and Olivier Fliche, director of the Fintech-Innovation division at the ACPR/Banque de France, opened the conference by raising some questions about the human control of algorithms : what is it for and where to place it in the governance loop of algorithms? How to make humans more independent from the machine? How will the AI Act organize human control?
As a first speaker, we had the pleasure to welcome Winston Maxwell, Director of Law and Digital Studies at Télécom Paris, Institut Polytechnique de Paris.
First, Winston highlighted the lack of consistency on the terms used for human control in European texts. For example, the AI Act speaks of « human oversight » in English versus « contrôle humain » in French, which is not exactly the same thing. The RGPD speaks of « significant human intervention ». He then presented his taxonomy of the different types of human oversight in two broad families: system oversight (of the entire algorithm) and individual oversight (of an algorithmic decision, such as a medical diagnosis).
Further, there are three purposes for which human control is needed: to detect errors, to ensure a fair and equitable process, and to establish accountability. He then cited the performance paradox as a barrier to effective human control: an algorithm is put into production if its predictive score is high, yet this can cause automation and accountability biases on the part of the human in charge of control.
Finally, Winston concluded his presentation with three recommendations for improving human control in the future: (1) distinguish the purposes of human control and for each, adopt a human control adapted to that purpose, (2) separate the tasks for the machine and for the human and differentiate the responsibilities (3) test the performance of the human-machine team for each purpose.
We then welcomed Thomas Baudel, Research Director and Master Inventor at IBM France R&D Lab.
We looked at the concrete case of filtering fraud alerts on financial transactions in which there is a real challenge to alleviate the task of humans with automated learning. Questions from different nature arise to implement an efficient human-machine collaboration. From an organizational point of view, the question of responsibility is paramount. Today, 85% of deployment projects fail because of unclear accountability mechanisms. From a legislative point of view, the AI Act emphasizes the respect of human autonomy. The technical point of view enables us to concretely determine the level of autonomy that can be granted to the machine. Finally, the economic and social sciences inform us about the definition of a responsible person, i.e. a person able to make an informed and uncoerced decision. Humans and machines complement each other in that humans can search for external information and can change decision criteria. Algorithms handle flexibility, exceptions, and novelty poorly while humans get tired and biased.
Finally, an IBM showed that if the algorithm has a performance below 70%, it would be counterproductive to use it. On the other hand, above 80%, the algorithm’s performance would be degraded by human intervention. IBM’s method of performance based on the algorithmic confidence makes it possible to justify investments in AI, to justify the use of AI to regulators, and to value human work in meaningful tasks in which they are more performant.
The last speaker of the conference was Astrid Bertrand, PhD student in AI explainability at Télécom Paris, Institut Polytechnique de Paris and at the ACPR’s Fintech-Innovation pole.
Astrid presented her work on explainability with the ACPR, including a study on robo-advisors in life insurance. In this context, explanations on why a certain contract is proposed for a certain client are required by financial regulations (Code des Assurances L.522-5 CdA). Yet, online robo-advisors often remain generic or evasive in these explanations, either because it is difficult to generate automatic explanations in real time or because the tools used are becoming increasingly complex, especially with the entry of AI.
In the presented study, a fictitious « explainable » robo-advisor was built, capable of generating automatic explanations for the reasons behind the advice. By having 256 participants test this robo-advisor, the study shows that the explanations given were counterproductive to the purpose of the regulation, which is to protect individual investors to ensure that they understand and are « empowered » to make choices. The explanations did not « empower » users; on the contrary, they could lead to overconfidence in unsuitable proposals.
Watch the replay (in French)
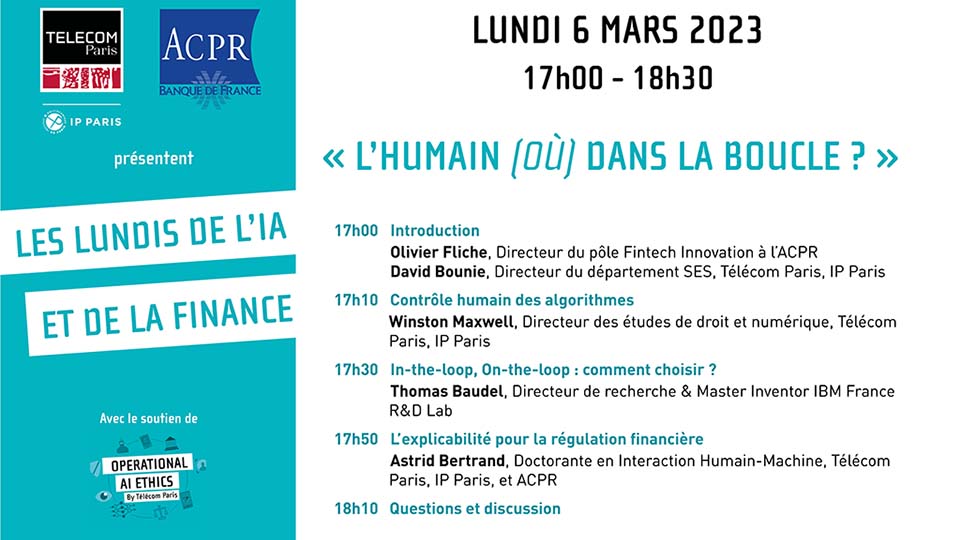