A paper about Few-Shot Emotion Recognition in Conversation accepted at EMNLP Conference
27 September 2021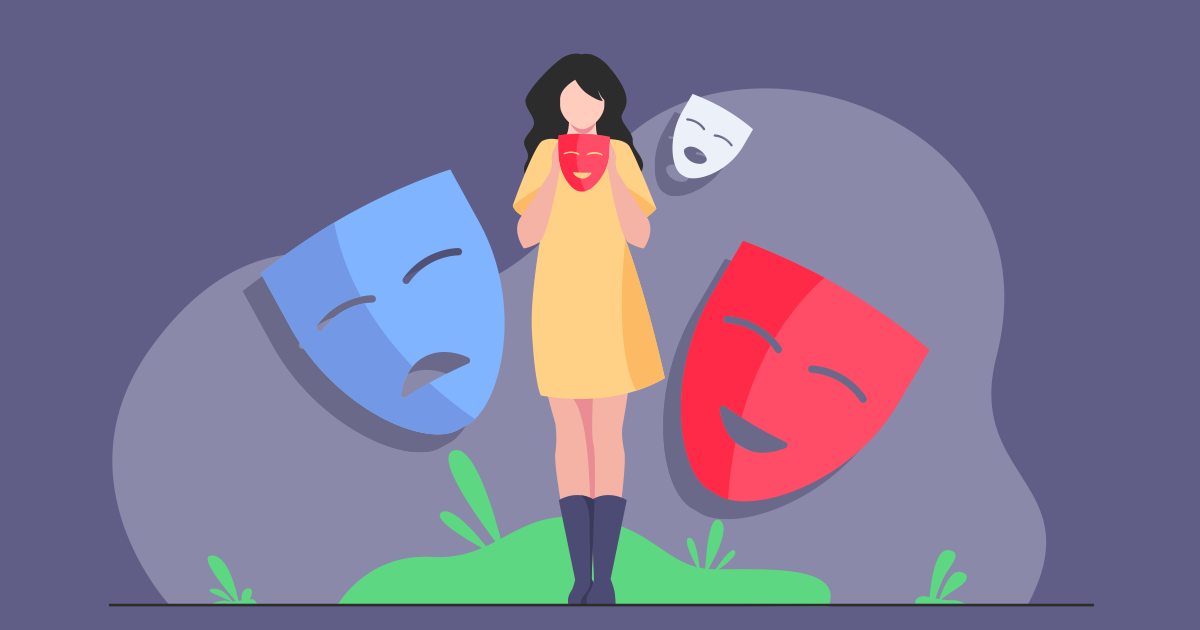
About the article
Several recent studies on dyadic human-human interactions have been done on conversations without specific business objectives. However, many companies might benefit from studies dedicated to more precise environments such as after sales services or customer satisfaction surveys. In this work, we place ourselves in the scope of a live chat customer service in which we want to detect emotions and their evolution in the conversation flow. This context leads to multiple challenges that range from exploiting restricted, small and mostly unlabeled datasets to finding and adapting methods for such context.We tackle these challenges by using Few-Shot Learning while making the hypothesis it can serve conversational emotion classification for different languages and sparse labels. We contribute by proposing a variation of Prototypical Networks for sequence labeling in conversation that we name ProtoSeq. We test this method on two datasets with different languages: daily conversations in English and customer service chat conversations in French. When applied to emotion classification in conversations, our method proved to be competitive even when compared to other ones.
Image source pch.vector/Freepik