Human Computer Interaction CHI 2023 Conference Highlights
22 May 2023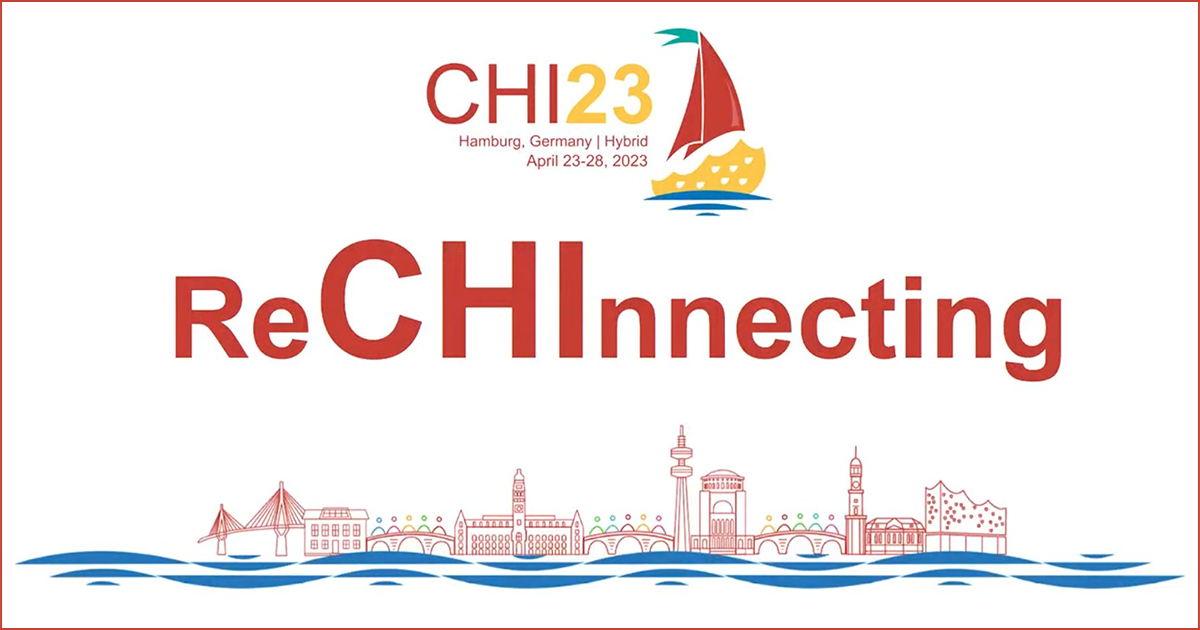
Our Operational AI Ethics team was able to present their work there. Astrid Bertrand presented her accepted paper on the different types of interactions in explainability interfaces, co-authored with Tiphaine Viard, Rafik Belloum, James Eagan and Winston Maxwell, which received an honorable mention. Other colleagues from the DIVA team (Design, Interaction, Visualization and Applications) in Télécom Paris, including Elise Bonnail, Wen-Jie Tseng and Samuel Huron also presented their accepted work on Virtual Reality and Data Physication.
The week was intense and packed with interesting talks and researchers; With a broad setting in Human-Computer Interaction, CHI makes a very fertile ground for interdisciplinary discussions; this was salient while looking at the sessions’ titles, which covered extended reality, social justice methodologies, poetry and art, as well as issues in artificial intelligence. Below are a few highlights noted by Tiphaine Viard, associate professor in AI & Society and Astrid Bertrand, PhD student at Operational AI Ethics.
Trying not to repeat societal biases in AI accountability
One such session was a panel on algorithmic accountability, which was held online by Daricia Wilkinson, and featured Deborah Raji, Ranjit Singh, Angelica Strohmayer, Ethan Zuckerman and Bogdana Ravoka. For 2 hours, the panelists discussed broadly about the main tenets of algorithmic accountability, focusing on ways to center the people who are typically marginalized in current society. The panelists highlighted the need for operational concepts (an approach we share at Operational AI ethics 🙂 ), and the need to take into account the broader social context (within patriarchy, capitalism, etc.) in order to avoid repeating the same mistakes over and over.
They stressed the need for a diversity of stakeholders in addition to interdisciplinary research, allowing situated points of views to be accurately taken into account, and advocated for a paradigmatic shift, from outcome-oriented systems to concern-oriented systems, that are able to react, integrate and acknowledge concerns and harms.
The subjectivity of the annotators who create datasets
In the research track, multiple sessions directly or indirectly discussed AI fairness. For example, Shivani Kapania (et al.) presented their study on the diversity of annotators that produce datasets, by conducting interviews of machine learning practitioners. The authors both linked their work to Genevieve Teil sociological notion of terroir, showing how ratings perceived as objective are situated within the practitioners’ frames of reference.
How explanations and ability to contest increases people’s fairness perception of the system
Another favorite of ours was “Disentangling Fairness Perceptions in Algorithmic Decision-Making: the Effects of Explanations, Human Oversight, and Contestability” , by Mireia Yurrita, et al. The speaker started her talk by referencing the CHI camera-ready submission interface, giving chills to the audience: the system would arbitrarily reject papers without any error message – there was no explanation, no human oversight, and no contestability. The speaker used this example to segue into her paper, which involved 267 participants that had to decide on the fairness of different fictitious credit scenarios. She discussed the different tension points that emerged in the study: between the amount of information and its understandability for all, between human involvement and timely decision-making, and between standardized fact-based processes and accounting for personal circumstances. A key finding was that some users would rather have a human overseeing their application, even in low-stakes contexts, whereas others preferred the lower processing and decision time. We are not the only ones who enjoyed this paper, since it got one of the best paper awards!
Enabling contestations of AI decisions by the public
Speaking of AI contestability, Kars Larsen from University of Delft, discussed the challenges with making AI contestable to the public, highlighting issues of representation of the civic participants, the lack of integration with existing democratic practices, and how to engage cities and local governments in the development of responsible AI: “Contestable Camera Cars: A Speculative Design Exploration of Public AI That Is Open and Responsive to Dispute”.
Decision-making with AI: groups make fairer decisions than individuals and framing explanations as questions improve people’s discernment
Many papers provided contributions on human’s decision-making process with AI and with AI explanations. For example, Chiang et al. investigated how groups of people made decisions based on AI’s outputs, compared to just one individual. They found that groups make fairer decisions, but rely more on AI, even when it is incorrect. However groups also are more confident when the AI is incorrect: Are Two Heads Better Than One in AI-Assisted Decision Making? Comparing the Behavior and Performance of Groups and Individuals in Human-AI Collaborative Recidivism Risk Assessment.
Related to the decision-making process with AI is the decision making process with explanations of AI. One problem is that people tend to blindly accept AI recommendations, especially when explanations are provided. To address this, Denry et al. found that framing explanations as questions “do you think x happened because of y ?” instead of causal facts “x happened because of y” forces people to reason better and make better decisions: Don’t Just Tell Me, Ask Me: AI Systems that Intelligently Frame Explanations as Questions Improve Human Logical Discernment Accuracy over Causal AI explanations.
Those are only a short selection of works we enjoyed – with 886 accepted papers this year, and 3800 attendees, there are many more in the proceedings!
Header image source Zhihan Zhang homes.cs.washington.edu