IP Paris Electrical Engineering Artificial Intelligence Day
From 9:00 am until 5:30 pm
The seminar will concentrate on the interplay between AI and the Electrical Engineering disciplines and show how this interdependency has the potential for decisive innovations.
During this one-day event, the current research in Artificial Intelligence and Electrical Engineering will be reviewed through plenary talks given by leading scientists and illustrated with examples taken from well-known laboratories as well as from the work of IP Paris teams.
The main topics that will be covered by the seminar are:
- AI and Information Theory
- AI and Communications
- AI in Electronics and Optics
The talks will cover the applications of AI to a number of various information environments. Questions such as implementation, speed, energy will be addressed. The talks will also examine the foundations of IA as well as its theoretical developments within the Electrical Engineering disciplinary fields.
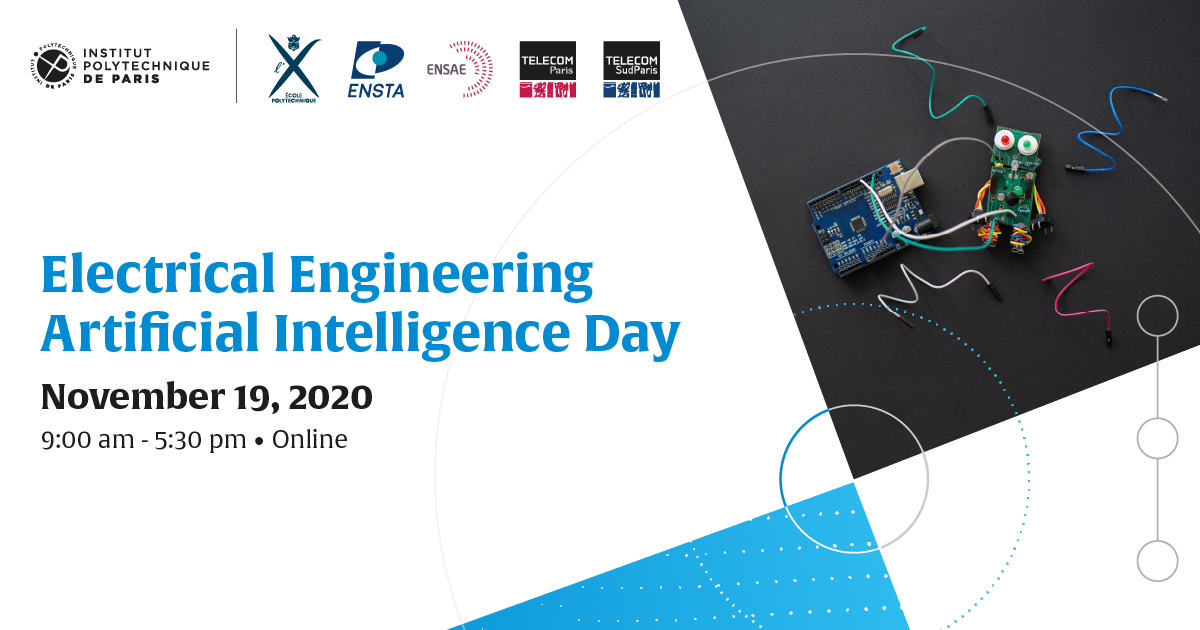
Program
09:00-90:10 Introduction
Bruno Thedrez, Head of the Information, Communications and Electronics (ICE) Department, Institut Polytechnique de Paris
Julie Grollier, Unité Mixte de Physique CNRS/Thales, Palaiseau
The advances in artificial intelligence are dazzling. Neural network algorithms are now able to perform better than humans on complex tasks, such as image recognition, or GO game. They are commercially used for their performance in big data analysis. As a main problem, they run on computers the architecture of which stands far away from the brain from which they are inspired. And in doing so, they consume a considerable amount of energy, four orders of magnitude greater than that of the brain, posing an environmental issue given their exponential use. To reduce such an energy payload and avoid incessant transfers of information at an unbearable energy cost, it would suffice to bring the memory closer to the calculation, as the brain does by entangling synapses and neurons. And why not, directly imitate synapses and neurons with electronic components and assemble them into circuits forming a neural network on a chip. This is the goal of neuromorphic computing.
Our brains are chemical and ionic. So, why designing electronic circuits? The only large-scale, miniaturized computing systems we know are based on electronics. They are capable of complex operations because they are the result of over seventy years of considerable industrial and academic efforts. If we are to realize brain-inspired systems in the years to come, a natural idea is to build on these advances, and base them on electronics. On the other hand, it must be complemented with new components and new functionalities to compactly and efficiently mimic synapses and neurons.
In this talk, I will describe the issues and challenges of neuromorphic electronics, recent advances in nano-synapses and artificial nano-neurons, as well as the developments expected in the coming years.
Julie Grollier is a researcher director in the CNRS/Thales lab in France. Her Ph.D. was dedicated to the study of a new effect in spintronics : the spin transfer torque. After two years of post-doc, first in Groningen University (Netherlands, group of B.J. van Wees), then in Institut d’Electronique Fondamentale (France, group of C. Chappert), she joined CNRS in 2005. Her current research interests include spintronics (dynamics of nanomagnets under the spin torque effect), and new devices for cognitive computation (in particular memristors).
Julie has over 100 publications, and is a frequent invited speaker in international conferences. She is also a Fellow of the American Physical Society. In 2010 she was awarded the Jacques Herbrand prize of the French Academy of Science, and in 2018 the Silver Medal of CNRS for her pioneering work on spintronics and brain-inspired computing. She is the recipient of two prestigious European Research Council grants: « NanoBrain » project (Memristive Artificial Synapses and their integration in Neural Networks, 2010-2015) and « BioSPINSpired » project (Bio-inspired Spin-Torque Computing Architectures, 2016-2021).
Julie is now leading the nanodevices for bio-inspired computing team that she initiated in 2009. She is also chair of the interdisciplinary research network GDR BioComp, coordinating national efforts for producing hardware bio-inspired systems.
Download the presentation | Watch the video
Sumanta Chaudhuri, ICE Department, Institut Polytechnique de Paris
In this talk I will focus on convolutional neural nets and their use in intelligent devices at the edge of the internet. Given their small power/energy budget, small form factors, and often low latency requirements, one has to resort to approximate methods. Approximation has an impact on both the architecture and the training algorithm of CNNs. Also both data approximation (quantization) and operator approximation (multiplier-less networks) will be discussed. I will present our work about a new type of multiplier-less network where all multiplications are replaced by a minimum operator and we will present experimental results on well known CNNs such as AlexNet, Cifar10.
Sumanta Chaudhuri obtained his PhD. Degree from ENST/ Télécom Paris in 2009. After two brief stints as post-docs in IEF Université Paris-Sud, and Imperial College London, he returned to Industry as a leading design engineer in Imagination Technologies UK, in 2012. Since 2014 he is a Lecturer/Researcher at Télécom Paris. His main research focus is on computer architecture, and physical implementation of computing.
Jean-Luc Danger, ICE Department, Institut Polytechnique de Paris
This talk gives first an overview of how the Machine learning techniques can be used for the security of embedded systems. It relies on concrete examples which are mainly to assess the power of physical attacks like side-channel attacks, fault injection attacks ou Hardware Trojan Horses detection. For a more more complex system like the autonomous car, the intrusion detection can take advantage of machine learning to check the integrity of dedicated IP protocols. The second part of the talk shows that the machine learning implementation can itself be the target of physical attacks and requires specific protections at design stage.
Jean-Luc Danger is full Professor at Télécom Paris / Institut Polytechnique de Paris. He is the head of the Secure and Safe Hardware research team whose main scientific topics are the security / safety of embedded systems and implementation of complex algorithms in ASICs or FPGAs. Jean-Luc authored more than 250 scientific publications/patents and co-founded the company Secure-IC. He received his engineering degree in Electrical Engineering from Supélec in 1981. After 12 years in industrial laboratories (PHILIPS,NOKIA), he joined Télécom Paris in 1993. His personnal research interests are randomness exploitation in digital circuits, protected architectures against cyberphysical attacks.
Download the presentation | Watch the video
11:00-11:15 Short break
Yann Frignac, Huawei Technologies
The recent increase of data acquisition, traffic and storage, as well as the need for massive data processing force the ICT research to explore new hardware architectures that will increase the efficiency in terms of velocity, energy and footprint.
As an example, new hardware systems and chips based on a neuromorphic design can match the need for highly efficient processing that exploits the capability of machine learning algorithms. Such hardware systems can offer an alternative solution to conventional GPU farms and datacenter the carbon footprint of which is an increasing concern.
In the quest for low energy, velocity and parallel processing, photonic systems and photonic integrated circuits are promising candidates. In this talk, a short overview of existing research paths toward this new goal will be discussed and supported by some implementation examples.
Yann Frignac received a Ph.D. in Electronic and Communications on fiber optics transmission system optimization from Telecom Paris and Alcatel CIT (now Nokia Bell labs). He joined Télécom SudParis in 2006 as an associate professor and became full professor in 2017. He joined Huawei Technologies France in 2020. His research domain focuses on optical fiber communication systems with projects on optical coherent technology, polarization and spatial multiplexing and large bandwidth optical amplifiers. He has contributed to a number of teaching programs in optical communications. He has recently shown interest for photonic approaches towards data processing and has initiated research actions in Institut Mines Télécom.
Download the presentation | Watch the video
Joe Wiart, ICE Department, Institut Polytechnique de Paris
The risk perception of electronic-magnetic field (EMF) exposure is nowadays a hot issue. The actual deployment of 5G infrastructures and base station antennas (BSA) strengthens such concern and reinforces the need for EMF improved exposure assessment and monitoring. The information relative to the RF sources of exposure is available in existing open databases (e.g. ANFR cartoradio), but information such as base station antenna locations and azimuth or frequency bands are never formally used to control or extrapolate the exposure measurements because of the related computational workload. To address the electromagnetic 3D simulation complexity, we are currently combining this information with the capabilities of Artificial Neural Network to perform « augmented » RF exposure measurements and build a performant EMF exposure mapping. In the presentation, we will develop these approaches and show our preliminary results.
Joe wiart is the holder of the Télécom Paris C2M chair dedicated to the characterization, modeling and control of exposure to electromagnetic waves. He is chairman of the “electromagnetics in biology and Medecine” commission of URSI as well as chairman on human exposure in CENELEC, the European Committee for Electrotechnical Standardization body. His field of expertise is numerical and experimental dosimetry as well as statistical and machine learning methods applied to the quantification of exposures. He has authored or co-authored more than 300 papers in International Journal and Conferences.
Download the presentation | Watch the video
Catherine Lepers, ICE Department, Institut Polytechnique de Paris
Data rate and energy consumption are the major challenges in optical networks. To reduce energy consumption, networks are becoming optically transparent, reducing O/E/O conversions in intermediate optical nodes. To face data rate increase, efficient use of optical fiber capacity and network resources without waste, is under consideration in new flexible and smart networks that cope with dynamic traffic.
Dynamicity and flexibility of the optical networks have been taken into account in new devices such as Reconfigurable Optical Add/Drop Mutiplexers (ROADMs). When WDM channels are added and/or dropped in ROADMs, wavelength dependent optical power excursion from Erbium Doped Fiber Amplifiers (EDFAs) evolves dynamically. To mitigate this impaiment, we have proposed to use machine learning methods to predict and to pre-compensate it. Furthermore, as physical layer impairments (PLIs) accumulate along the path, we have considered optical power excursion together with optical signal to noise ratio (OSNR) and bit error rate (BER), to estimate QoT of unseen channel configurations using reinforcement learning approach.
Catherine Lepers received the PHD degree (Chaotic Dynamics in Lasers) from University of Lille, France, in 1993. She is Full Professor with the department of Electrical Engineering and Dean of the Faculty at Institut Polytechnique de Paris/ Telecom SudParis. She is the Head of the Optics and Photonics Group in her department. Before joining IP Paris/ Telecom SudParis, she was an Associate Professor at University of Lille where she conducted research on Dynamics in Lasers and Photonics. From 2000 to 2008, she performed research on OCDMA in optical communications at IP Paris/ Telecom Paris as associate researcher.
Her present research interests in SAMOVAR Lab. include machine learning for optical networks and visible light communications. She managed projects devoted to home networking, ROADM node evaluation and multilayer network dimensioning. She supervised a MOOC on Optical Access Networks.
She is a full member of the Light Communications Alliance (LCA). She is Deputy Head of the research committee in DIGICOSME Labex. She represents Telecom SudParis in Information Communication and Electronics Domain at Doctoral School of IP Paris. She is a member of the Academic Council from IP Paris.
Download the presentation | Watch the video
12:30-14:00 Lunch break
Deniz Gündüz, Imperial College London
This talk will be on the interplay between machine learning and wireless communications. In the first part of the talk I will show how machine learning can help improve wireless communication systems. Communication system design traditionally followed a model-based approach, where highly specialized blocks are designed separately based on expert knowledge accumulated over decades of research. I will show that data-driven end-to-end designs can meet or even surpass the performance of these highly optimised block-based architectures. In particular, I will focus on wireless image transmission, and show that deep learning based joint source-channel coding architecture not only outperforms state-of-the-art digital communication systems based on separate image compression (BPG/ JPEG200) followed by near capacity-achieving channel codes (LDPC), but also provides graceful degradation with channel quality and adaptation to bandwidth. In the second part of the talk, I will focus on federated learning across wireless devices at the network edge, and show that jointly designing the communication protocol with the learning algorithm significantly improves the efficiency and accuracy of distributed learning across bandwidth and power limited wireless devices. In both parts of the talk I will highlight the convergence of machine learning and wireless communication system design, and point to some promising new research directions.
Deniz Gündüz received his M.S. and Ph.D. degrees in electrical engineering from NYU Tandon School of Engineering in 2004 and 2007, respectively. After his PhD, he served as a postdoctoral research associate at Princeton University, and as a consulting assistant professor at Stanford University. He was a research associate at CTTC in Barcelona, Spain until September 2012, when he joined the Electrical and Electronic Engineering Department of Imperial College London, UK, where he leads the Information Processing and Communications Lab. His research interests lie in the areas of communication and information theory, machine learning and privacy. Dr. Gündüz is an Editor of the IEEE Transactions on Wireless Communications and the IEEE Transactions on Green Communications and Networking. He also served as a Guest Editor for the IEEE Journal on Selected Areas in Communications Special Issue on “Machine Learning for Wireless Communications”, and as an Editor of the IEEE Transactions on Communications (2013-2018). He is the recipient of the IEEE Communications Society – Communication Theory Technical Committee (CTTC) Early Achievement Award in 2017, a Starting Grant of the European Research Council (ERC) in 2016, IEEE Communications Society Best Young Researcher Award for the Europe, Middle East, and Africa Region in 2014. He has received Best Paper Awards at GlobalSIP 2019, WCNC 2018, WCNC 2016 and ISIT 2007. He is a Distinguished Speaker of the IEEE Information Theory Society (2020-21)
Download the presentation | Watch the video
Emilio Calvanese-Strinati, CEA LETI
This talk promotes the idea that including semantic and goal-oriented aspects in future 6G networks can produce a significant leap forward in terms of system effectiveness and sustainability. Semantic communication goes beyond the common Shannon paradigm of guaranteeing the correct reception of each single transmitted packet, irrespective of the meaning conveyed by the packet. The idea is that, whenever communication occurs to convey meaning or to accomplish a goal, what really matters is the impact that the correct reception/interpretation of a packet is going to have on the goal accomplishment. Focusing on semantic and goal-oriented aspects, and possibly combining them, helps to identify the relevant information, i.e. the information strictly necessary to recover the meaning intended by the transmitter or to accomplish a goal. Combining knowledge representation and reasoning tools with machine learning algorithms paves the way to build semantic learning strategies enabling current machine learning algorithms to achieve better interpretation capabilities and contrast adversarial attacks. 6G semantic networks can bring semantic learning mechanisms at the edge of the network and, at the same time, semantic learning can help 6G networks to improve their efficiency and sustainability.
Dr. Emilio Calvanese Strinati obtained his Ph.D in Engineering Science in 2005 from Telecom Paris, France. He worked at Motorola Labs between 2002 and 2006. In 2006, he joined CEA LETI as a research engineer. From 2007, he becomes a PhD supervisor. From 2011 to 2016 he was the Smart Devices & Telecommunications European collaborative strategic programs Director. Between December 2016 and January 2020, he was the Smart Devices & Telecommunications Scientific and Innovation Director. In February 2018, he directed the first 5G mobile millimeter waves demonstration in realistic operational environments at the 2018 winter Olympic Games, 5G technologies. Since 2018, he holds the French Research Director Habilitation (HDR). Since February 2020, he directs activities at CEA LETI focusing on future 6G technologies. E. Calvanese Strinati has published around 120 papers in international conferences, journals and books chapters, given more than 150 international invited talks, keynotes and tutorials. He is the main inventor or co-inventor of more than 60 patents.
Download the presentation | Watch the video
Mireille Sarkiss, ICE Department, Institut Polytechnique de Paris
This talk focuses on efficient transmission techniques for an energy harvesting (EH)-enabled mobile device with offloading capabilities to a nearby base station at the network edge, which can be endowed with more computation resources. The objective is to propose policies that jointly optimize resource scheduling and computation offloading under strict delay constraints. Such problem can be formulated as a Markov Decision Process and optimal offline policies based on Dynamic Programming (DP) approaches are studied to resolve it. However, model-based DP approaches become impractical when the dynamic environment becomes complex and the system states large. In order to overcome such constraint, we propose to investigate function approximation techniques via deep neural networks leveraging on Deep Reinforcement Learning (DRL) algorithms.
Mireille Sarkiss received the engineering degree in Telecommunications and Computer Science from the Lebanese University, Faculty of Engineering, Lebanon, in 2003 and the M.Sc. and Ph.D. degrees in Communications and Electronics from Telecom Paris, France, in 2004 and 2009, respectively. She was a doctoral researcher at Orange Labs from 2004 and 2007 and a post-doctoral researcher with the Department of Communications and Electronics, Telecom Paris, from 2009 to 2010. From 2010 to 2018, she was a Researcher with CEA LIST, Communicating Systems Laboratory, France. In 2018, she joined the Communications, Images and Information Processing Department, Telecom SudParis, France, as an Associate Professor. Her research interests include coding and decoding, resource allocation, physical layer security and distributed hypothesis testing for wireless communications.
Download the presentation | Watch the video
15:50-16:05 Short break
Jakob Hoydis, Nokia Bell Labs
Machine learning (ML) starts to be widely adopted in the telecommunications industry for the optimization and implementation of the fifth generation of cellular networks (5G). However, no component of 5G has been designed by ML. In this talk, I will describe the idea of and road towards a possible 6G system which is designed in a way that ML is given the opportunity to design parts of the physical and medium access layers itself.
Jakob Hoydis is currently head of a research department at Nokia Bell Labs, France, focusing on radio systems and artificial intelligence. Prior to this, he was co-founder and CTO of the social network SPRAED and worked for Alcatel-Lucent Bell Labs in Stuttgart, Germany. He received the diploma degree (Dipl.-Ing.) in electrical engineering and information technology from RWTH Aachen University, Germany, and the Ph.D. degree from Supéléc, Gif-sur-Yvette, France. He is a co-author of the textbook “Massive MIMO Networks: Spectral, Energy, and Hardware Efficiency” (2017). He is currently chair of the IEEE COMSOC Emerging Technology Initiative on Machine Learning, Editor of the IEEE Transactions on Wireless Communications, as well as Area Editor of the IEEE Journal on Selected Areas in Communications Series on Machine Learning in Communications and Networks.
Download the presentation | Watch the video
Ghaya Rekaya, ICE Department, Institut Polytechnique de Paris
Lattices are impressive mathematical objects that have many important applications such as in integer linear programming algorithms to factorize polynomials with respect to rationals; in cryptographic algorithms that realize very expressive features like fully homomorphic encryption; and in the shortest vector problem which consists in finding the closest vector in a random lattice chosen from a distribution. Among the various lattice problems, many remain open. In this work, we focus on the problem of counting lattice points in an n-dimensional sphere. The number of lattice points inside the sphere is proportional to its volume. However, we do not know the density of lattice points inside a given sphere. This is an interesting problem in pure geometry and it has considerable practical importance, especially when the lattice dimension is large. This problem is deeply related to complexity theory, in particular to the closest vector problem (CVP), and to the Fincke and Pohst variant, which have been used for decoding in wireless MIMO communication systems as the sphere decoder (SD) algorithm. This problem is known to be NP-hard and not tractable. In this work, we propose a modified SD algorithm by introducing a systematic approach to the design and control of the sphere radius based on deep neural networks (DNN). The learning model is introduced to predict the number of network points within the sphere having a certain radius. Since this number is intelligently learned by a DNN, the SD updates the radius until a small number of points are expected and then begins the search phase. We show by simulation that for high-dimensional MIMO systems, the number of lattice points is strongly reduced in the new SD algorithm, leading to a complexity only 3 times higher than that of the MMSE decoder.
Ghaya Rekaya-Ben Othman is a professor at Télécom Paris, Institut Polytechnique de Paris. Her research focuses on cutting-edge topics in the field of telecommunications, including MIMO/Massive MIMO systems, coding and security for Physical Layer Networks Coding and for massive space division multiplexing (SDM) fiber optic communications. She has achieved benchmark results such as the Golden Code and has also contributed significantly to the technological convergence between wireless and optical communications. Her research work has resulted in more than 100 publications in international journals and conferences and the filling of more than 40 patents. Technological innovation is at the heart of her activities, both in teaching and research. She is a trainer in « technological innovation » for engineering and doctoral training. She received the prize of the City of Paris for the best young woman scientist in 2007. She received the best paper award of the International Conference on Communication and Networks (COMNET) in 2018. She was named « Chevalier dans l’ordre des Palmes Académiques » in January 2020.
Download the presentation | Watch the video
16:55 Concluding remarks